Every day we wake up, and before engaging with another human being, we either passively or actively engage with an algorithm connected to a sensing system. These ‘thinking systems’ acquire, interpret and measure our presence – our interaction with the physical world, our biological information and the way we feel.
They regulate, in some fashion, almost every aspect of our daily lives and form a critical component of the ecosystems designed to deliver goods and services anytime, anywhere.
To meet a globalized demand for near-instant access to goods and services, organizations heavily instrument each component of their inordinately complex supply chains. They leverage machine learning (ML) models or trained algorithms to make decisions geared toward increasing efficiencies while maintaining quality and customer satisfaction.
Humans are a part of these supply chain processes, and automated systems are increasingly being used to monitor and supervise workforces, often emulating the functions of human managers, instructing people on how to conduct themselves before, during and after their job. To increase productivity, employee and workforce monitoring systems move to collect more biometric information to boost employee engagement and improve safety.
US legal and regulatory frameworks have recently been established to help regulate the future of algorithmic work. For example, California just passed a law designed to govern and regulate transparency, fairness and safety around warehouse quota or monitoring systems. While this law deals specifically with warehouse distribution centers, as more algorithms are used to manage workforces, people’s day-to-day activities, and their pay, there will be increased scrutiny across the board.
The rise of algorithmic work regulations is forcing enterprise GRC capabilities to rethink their ML models’ risk and control structures, starting with making them explainable.
Explainable AI (XAI) is the concept that an ML model and its output must, at every single stage, be explained in a way that is interpretable or understandable to an average person. Making ML models explainable isn’t just a reaction to a regulatory requirement; XAI improves the end-user experience of a product or service, increases trust and ultimately improves quality.
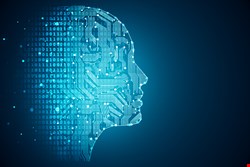
Regulatory agencies increasingly require companies to disclose more about these systems to combat the perception that algorithms are black-box systems that cannot be explained.
The Information Commissioner’s Office (ICO), a UK-based independent authority for data privacy, and The Alan Turing Institute partnered to conduct extensive research around making AI explainable. They have devised a framework that describes six different types of explainability:
- Rational explanation: What were the reasons that led the system to arrive at its decision?
- Responsibility explanation: Who are all the team members involved in designing, developing, managing and implementing an AI system? This includes defining the contact information for requests to have a human review an AI-driven or assisted decision.
- Data explanation: This explanation is critical. Here we document what data was used in a decision and how. This includes a description of the training datasets.
- Fairness explanation: This is where we discuss bias. The fairness explanation looks at the design and implementation of an AI system to ensure that its decisions are unbiased and fair and the individual has been treated equitably.
- Safety and performance explanation: What are the steps taken across the system that maximize the accuracy, reliability, security and safety around its decisions and behaviors?
- Impact explanation: What are the controls in place to both consider and monitor the impacts that the use of an AI system and its decisions has or may have on an individual, the workforce or even broader society?
While building an explainable system, it is vital that we adhere to a set of guiding principles. Therefore, ICO leveraged the principles outlined in GDPR as inspiration for the four principles for making an AI system explainable:
- Be transparent: Fully and truthfully documented processes around your company’s use of AI-enabled decisions; when and why.
- Be accountable: Employees who govern the oversight of the “explainability” requirements of an AI decision system must ensure that explainability requirements are present in the design and deployment of the models.
- Consider context: When planning on using AI to help make decisions about your workforce, you should consider the setting in which you will do this, the sector and use case contexts and the contextual factors around how you would deliver explainability to the impacted users.
- Reflect on impacts: Build and deploy your ML system to consider impacts in areas such as physical, emotional, sociological, impact on free will, privacy and implications on future generations
While understanding the principles and types of explainability are a good starting point, cybersecurity and GRC workforces must increase their competence in auditing, assessing, protecting and defending artificially intelligent systems. We have quite a way to go.
This year, ISACA issued cornerstone studies on the state of cybersecurity and GRC workforces. These studies highlighted challenges, including a widening skills gap, issues in gaining access to a pipeline of qualified applicants and budget reduction. As security and GRC organizations must be responsive, we have turned to AI to help mitigate our risks.
Key Highlights
- The use of ML or robotic process automation/RPA in security operations is increasing. Roughly 34% of the respondents stated that they use AI in SecOps – up four percentage points from a year ago.
- Over a fifth (22%) of the respondents have increased their reliance on artificial intelligence or automation to help decrease their cybersecurity skills gap. This compounds the issue as we lack the skills to govern, protect and defend these new AI systems.
- While these technologies are not yet replacing our human resources, they may instead shift the types of resources required. For example, AI may broadly decrease the number of analysts needed; however, human resources will be reallocated to designing, monitoring and auditing algorithms.
"We must begin building and training on AI risk, controls, and audit frameworks, and train our personnel in the field"
Given that GRC and cybersecurity organizations have the mandate to govern, assess, protect and defend both enterprise use of AI/ML systems and our own use in areas such as SecOps, we must begin building and training on AI risk, controls and audit frameworks, and train our personnel in the field.
Key steps to help GRC professionals establish a framework for auditing and assessing compliance around algorithms include:
- Document all six explainability types for your algorithm. What are the processes that streamline the complete transparency and accountability of your AI model? Describe the setting and industry in which the AI model will be used and how this affects each of the six types of explainability.
- Document all data collection and preprocessing activities. How representative is the data about those impacted by the AI system? Where was the data obtained, how was it collected and is it aligned with the purpose for which it was initially collected? Is the system using any synthetic data? How was it created? Do any of the datasets involve any protected characteristics? Did the team detect any bias or determine if the involved data sets reflect past discrimination? How did they mitigate it?
- Assessment of the entire AI team’s diversity. Was the team that was involved in the system design diverse? Did the team reflect the user population the algorithm serves? Was there anyone on the team who is neurodiverse? Was there an evaluation to determine if a more diverse team would design a system more resilient to bias?
- Assess all documentation, processes and technology in place to ensure the system is built to extract relevant information for explainability. Is the system explainable by design? In selecting the AI model, did the team consider the specific type of application and the impact of the model on decision recipients? What is the total cost of ownership for the AI system, and is it cheaper than the previous potentially more explainable system? For systems leveraging social, identity or biometric information, did the team seek to make interpretability a key requirement? If the organization has chosen to leverage a 'black box' system, did the team document the rationale? How are the models tested, and does the change management process include model updates and versioning records? Who is responsible for validating the explainability of our AI system?
- Document and validate the team’s rationale for the AI system’s results. How is the team visually representing the logic of the system’s output? What tools are being used to present the results in a way that is interpretable to our workforce?
- Define how your organization prepared implementers to deploy the AI system. How has your organization trained your AI system implementers? Can they detect where bias may occur and how to mitigate it?
- How did the organization incorporate security into the design? Did the organization perform a system-level risk assessment? Is there a risk and control matrix for the ecosystem? Did the team create a threat model and define the application and ecosystem security requirements? How was the system penetration tested? What was the secure code review process and what tools were used? What were all the types of attacks identified, and what security logging, monitoring and defense patterns were created? Can the defense systems successfully detect AI/ML system-specific attacks – for example, data poisoning attacks? What are the incident response and forensics processes and playbooks should the system be breached?
- Document the roles and responsibilities in the development of your algorithm. For example, strategist, product manager, designer, architect, AI development team, implementer, AI operations team, security and compliance, senior and executive management. Was everyone adequately trained?
- Define and review all subjects that documentation needs to reflect. For example, the decision to design the system, describing the explanation types and how the principles were applied, data collection and acquisition process, data preprocessing, model selection, building, testing and monitoring. What are the tools used for selecting an explanation and how explanations will be delivered to requestors? What is the compliance policy, the risk and control matrix and the entire security plan for the AI system?
As more laws pass that force organizations to be transparent, AI systems must be designed for explainability before the first line is written. Remember, if the team is unable to explain the entire system in easy-to-understand terms, the system is not well designed.
Key References
We leveraged co-badged guidance by the ICO and The Alan Turing Institute, which aims to give organizations practical advice to help explain the processes, services and decisions delivered or assisted by AI to the individuals affected by them.
- The Basics of Explaining AI: Part 1
- Explaining AI in Practice - Part 2
- Explaining Ai Decisions: Part 3
Examples of laws and regulations around AI
- California Assembly Bill No. 701 - Warehouse Distribution Centers
- GDPR (Articles 5, 13, 14, 15, 21, 22, 35)
- Parts 3 and 4 of the DPA 2018
- Equality Act 2010
- California Assembly Bill AB1215 - Law enforcement: facial recognition and other biometric surveillance
- Illinois Artificial Intelligence Video Interview Act (AIVIA)