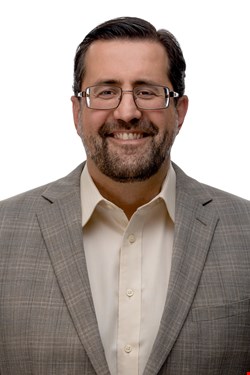
The role that machine learning/artificial intelligence (ML/AI) is playing in the security of data has become one of the most talked about subjects in the cybersecurity industry over the last couple of years.
With the sector continuing to battle against evolving cyber-threats, handle the exponential growth of technology and maintain productivity amid an ongoing skills shortage, there are many turning to autonomous, cognitive computing solutions to support them in their efforts to keep information safe.
At the same time, the malicious use of this technology has also become more apparent, and Infosecurity recently spoke to Oliver Tavakoli, CTO of Vectra Networks, to get his perspectives on how cyber-criminals are using and abusing ML/AI in their attacks, to what effect and whether there is a way to make it more secure.
How much are cyber-criminals using AI and ML in their attack strategies?
It’s difficult to know for sure. AI and ML are tools which can be used for automation and which can enable customization of attacks in ways which would have been considered impractical just a few years ago, but the defensive side of cybersecurity tends to see the end product of such attack campaigns and it is hard to definitively determine from an individual attack if any ML went into its creation.
However, there are signs that the same techniques which Cambridge Analytica applied to the US election and which marketing teams use to improve click-through rates on emails are also being utilized to craft more targeted phishing campaigns. As an attacker, if I can craft an email that takes into account all sorts of data about you available on social media, you’re more likely to believe that it is authentic.
There is no sign that attackers are putting AI in charge of autonomously coordinating an entire campaign; most goals the attackers have rely on stealth rather than speed. However, there will clearly come a time when defenders will get better at detecting stealthy attacks – at that point, speed will warrant a premium and a sufficiently sophisticated AI may need to be given the keys as both attackers and defenders escalate their tactics. A glimpse of this future can be seen at the Cyber Grand Challenge.
"AI and ML can be applied to automate a series of problems attackers have and to increase the likelihood of success in their business models"
What could attackers gain by harnessing AI/ML?
AI and ML can be applied to automate a series of problems attackers have and to increase the likelihood of success in their business models. For example, ML can be used to divine the limits of existing preventive technologies and AI can be used to craft more effective phishing campaigns which have much higher click-through rates.
It can also be utilized for automating many elements of vulnerability discovery and exploit creation and to automate discovery of how to slip past existing detection technology. There are very few individual aspects of what attackers do which are not candidates for the type of automation which can be achieved through the use of AI and ML techniques.
What should companies consider when using/buying a machine learning service?
Most companies don’t really buy a ‘machine learning service’. They usually end up being interested in solutions which are produced by vendors or service providers who claim to harness ML to create those solutions. Companies should carefully study the threat models they are concerned about, and test the products or services which claim to protect against them.
Once a company has thoroughly vetted the proposed solution with a realistic test simulation, it should engage the solution provider to understand how they use ML – what inputs do they require, how do they train their algorithms, how often does the algorithm have to be updated etc. in an effort to understand whether attackers could easily learn to circumvent the solution.
Many companies are, of course, looking to apply ML techniques to a wide variety of business processes. The companies who have the largest commitment to hiring data scientists have even chosen to apply some of them to cybersecurity use cases. Attempts to marry the disciplines of cybersecurity and data science have, to date, either ended in initial failures or in a failure to sustain the initial modest successes. Most companies are not set up to build and maintain and rapidly evolve such a solution.
How can the use of machine learning be made more secure/trustworthy?
It can’t. Think back to when cryptography first became prevalent for securing computer communications. The goal was simple – to prevent attackers from being able to gain access to confidential data. Of course, cryptography also became a tool of choice for attackers – to prevent defenders from being able to eavesdrop in on their communications or analyze elements of their attacks.
ML is knowledge – and like all knowledge, it is a genie which cannot be put back in the bottle. Each side in this battle will utilize ML for its own purposes and ML has such broad applications beyond cybersecurity that the knowledge and tools will continue to evolve, absent investment in it by cybersecurity companies. Throughout the history of humankind, knowledge has been used for noble and nefarious purposes. ML, like most knowledge, is itself neutral.