The concepts of machine learning and artificial intelligence (AI) have grown to become almost synonymous with information security and the protection of data – with more and more enterprises turning to automation and ‘cognitive computing’ to improve the proficiency of their security efforts. Such tech provides quicker response times, better threat detection, the ability to process and analyze large amounts of data and can free up vital staff time.
However, where there is light there is also dark. Cyber-criminals are constantly looking for the next best, and quickest, way to carry out attacks to the highest impact. According to recent research from ESET, the threat of AI being used as a weapon against organizations has led to a significant amount of IT decision makers (75%) in the US to believe that the number of attacks they have to detect and respond to will increase.
While this fear is lessened among their European counterparts, with 57% in the UK and 55% in Germany concerned about AI-attacks, the worry still exists. What’s more, 71% of IT decision makers surveyed believed AI will make attacks more complex.
Where Hackers Use AI
According to Corey Nachreiner, CTO at WatchGuard Technologies, as machine learning and AI is still less than a decade old in security, more often he sees good researchers showing the potential risks in how attackers might misuse machine learning/AI, than he does attackers actually exploiting it in the real world.
“It is not unusual for the good guys to notice potential risks before the bad guys start using them and we probably have to wait a year or two before attackers really start leveraging machine learning for attacks,” he says.
“On the other hand, the malicious use of machine learning/AI might not always be immediately apparent. For instance, if an attacker used machine learning to improve the efficacy of phishing emails, all the real world would see is a well-crafted email. It would be hard to know if that change was a result of applying machine learning algorithms to perfect phishing.”
He adds that there is at least one company, Darktrace, which claims to have detected attackers using machine learning to learn a victim’s network behavior, although he admits he hasn’t seen the evidence to support that himself.
“If an attacker used machine learning to improve the efficacy of phishing emails, all the real world would see is a well-crafted email”
Likely Attack Vectors & Greater Sophistication
Attackers are already using automation, so by adding some ‘intelligence’ to that automation, they get more powerful and become more effective, according to Cesar Cerrudo, CTO of IOActive, a cybersecurity consultancy.
“Currently many attacks are just blind and hitting everything until they hit something vulnerable, with a bit more intelligence attackers can increase attack effectiveness and success rate,” he says. “For instance, instead of trying to blindly attack a Linux system with a Windows exploit, which of course won’t work, attackers could know exactly what systems they are attacking, what system version, language, time zone etc. and also when they should attack and how they should do it.”
He adds that this means they can craft specific, targeted attacks and scale all of this in an easy way. “For example, by having enough data on targeted systems you can profile a company on how long it takes them to patch systems and how often they do it so when there is a new vulnerability they will know what companies could remain vulnerable and for how long, prioritizing what systems to target first.”
According to Elliot Rose, head of cybersecurity at PA Consulting, AI systems suffer from several unresolved vulnerabilities which criminals can exploit to create new opportunities for attacks.
“Machine learning algorithms like those in self-driving cars create an opportunity to cause crashes by presenting the cars with misinformation. Military systems could also be misled in a way that could lead to a friendly fire incident,” he says.
He adds that AI systems are susceptible to attacks in a number of ways. “Data poisoning introduces training data that causes a machine learning system to make mistakes,” says Rose. “Adversarial attacks provide inputs designed to be misclassified by machine learning systems such as teaching an autonomous vehicle to misclassify a stop sign. Attackers can also exploit flaws in the design of autonomous systems’ goals.”
Rose warns that AI-enabled impersonation is a new threat to systems that can mimic individual voices. “Significant progress in developing speech syntheses that learn to imitate individuals’ voices opens up new methods of spreading disinformation and impersonating others,” he explains.
“AI could automate the identification of suitable targets, research their social and professional networks, and then generate messages in the right language”
Spear Phishing
Just as AI speeds up legitimate activity, it creates opportunities for criminals to increase the effectiveness of their attacks. According to Rose, spear phishing attacks which use personalized messages to extract sensitive information or money from individuals require a significant amount of effort and expertise.
“AI could automate the identification of suitable targets, research their social and professional networks, and then generate messages in the right language. This could enable the mass production of these attacks. AI could also be used to increase the speed of attackers in identifying code vulnerabilities and trends,” he says.
Nachreiner adds that two years ago a team gave a talk on ‘Weaponizing Data Science for Social Engineering’ showing how they used a neural network to create an automated Twitter phishing bot.
“We are not seeing this in real attacks yet, but it is coming. Also, you may not know whether an improvement to an attacker’s malware or emails is due to their individual improvement or machine learning solutions,” he warns.
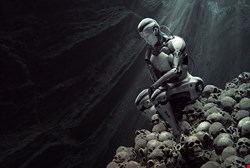
Tampering with AI Systems
Not only are hackers using AI to attack organizations, they are targeting organizations’ own AI and machine learning systems and infrastructure.
Nick Dunn, managing security consultant at global information assurance firm NCC Group, says that although the attack surface consists of the same components as traditional systems, including hardware, data collection pipelines, local storage and cloud storage, organizations are seeing a rise in the number of bespoke attacks being launched against them that involve the compromise of the learning data and learning process.
“The level of interaction in machine learning systems between these components and back-end data is becoming an increasingly viable route for hackers to exploit, especially when voice recognition and fraud detection features play a part in the system,” he says.
He adds that the attacks are usually carried out through manipulation of data inputs to systems during either the training stage or the operation phase.
“Whether these are intended to modify or harvest data, there’s no doubt that attackers are looking to cause significant damage,” says Dunn.
It’s also important to be aware of issues arising from the opacity of systems and any legal issues associated with this, according to Dunn. The complex nature of algorithms can create situations where it is not immediately clear that a system is giving an incorrect output, while legally-enforced removal of training data can result in the system no longer functioning correctly or becoming redundant, he says.
Dunn argues that securing machine learning software during the Software Development Life Cycle (SDLC) forms a significant foundation in terms of mitigating threats facing software. Key IT stakeholders across a business need to be aware of threats and the ways in which these can be mitigated from the get-go. “Resilience is also an important consideration, as systems may need to be restored or modified following the removal or correction of training data.”
Defending Against AI Attacks
Cerrudo says that organizations need to keep defending as usual but focus on becoming faster and more proactive since, with new technologies, attackers get faster and better. “For instance, they could instantly know when a system becomes vulnerable and attack it. So as an organization you will have less time to apply patches to get your systems secure,” he says.
Far from using AI to beat the hackers using AI themselves, Rose says that humans will still be needed to keep systems secure.
“The big technology companies have started recruiting aggressively for experts in the past six months. We need security algorithms to be less of a black box so we can spot deviations in how they work. We also need humans as a check when processing sensitive or personal data, such as data on sexual orientation, health or children,” he says.
Governments will also need to step up and play a role in protecting infrastructures from AI-based attacks. “We know that many of the tools used in cyber-attacks come from countries such as Russia and they will be using AI to improve these. The UK needs to think how it can use AI to better defend against such attacks by spotting trends early and providing solutions and guidance,” says Rose.
Future AI-Based Attacks
Hackers aren’t investing that much in the R&D of AI and machine learning since they tend to do the least effort to get the maximum profit, Cerrudo says.
“Once, however, commercial and open source AI and machine learning software becomes more available and easier to use, cyber-criminals will start to use it for sure.”
Nachreiner says although few cyber-criminals leverage machine learning/AI currently, that doesn’t mean it won’t happen. “Believe it or not, cyber-criminals are usually behind information security researchers,” he says.
There are many new attacks and techniques highlighted at conferences like DEF CON and Black Hat long before any criminal attacker weaponizes them, according to Nachreiner. “The fact that cyber-criminals haven’t widely started leveraging machine learning yet doesn’t mean it’s not an upcoming threat, but it does allow you to prepare for these attacks before they happen.”
While it is hard to predict exactly when criminals will be widely using AI to mount attacks, organizations must get ready and ensure that the appropriate counter measures are in place so as to mitigate the impact as much as possible.