If the question is how to control harmful content, Davey Winder considers whether the answer is machine learning?
The proposed introduction of new safety features from Apple to address the problem of child sexual abuse material (CSAM) erupted into a heated privacy debate. At the heart of this discussion was the use of ‘on-device’ machine learning (ML) to warn about harmful content within the iMessage app.
ML, a branch of artificial intelligence (AI) that essentially mimics human learning but with data and algorithms, is increasingly used for monitoring and controlling digital content. Infosecurity investigates just how advanced it really is.
Far From Infallible
The obvious starting point for any such investigation has to be an understanding of exactly how advanced and reliable ML is in this regard right now. Dr Sohrob Kazerounian, head of AI research at Vectra AI, has worked with the Centre for European Policy Studies (CEPS) to offer recommendations on EU policy on AI. Dr Kazerounian does not think there’s a definitive answer to these questions currently. “Even as datasets to test algorithms against become more common, much of our understanding of how ML performs in real-world scenarios comes from self-reporting on data that is not readily available to scrutiny,” he says. Another and perhaps more pernicious problem is that humans can’t seem to agree amongst themselves about what is, or isn’t, harmful content or misinformation, according to Dr Kazerounian. “This is critical because ground-truth labels from humans are necessary to train ML systems to distinguish harmful from non-harmful content, or misinformation from truth.”
The latter being particularly pertinent when, as Dr Preethi Kesavan, head of the school of technology at the London School of Business and Finance Singapore (LSBF), points out, “several tools with AI capabilities include humans for decision-making and detecting and controlling harmful content and misinformation.” The algorithms allow people to handle more cases, she says, while prioritizing material and eliminating tedious tasks that consume more time. “Most of the ML technology that is successfully implemented use the Naive Bayes algorithm,” Dr Kesavan continues, “and most of the prevailing harmful content is collected via chatbots between the victim and the perpetrator.” However, here lies the rub, as CEO of Edge-AI surveillance solutions company Digital Barriers, Zak Doffman, says, “ML is far from infallible and is only ever as good as its training data and a setup where its ‘learning’ can be continuous.”
Move this into the realm of harmful images and beyond the straightforward matching as seen with cloud-based CSAM filtering, and you will find a complex problem to solve. “Looking for something interpretative like ‘sexually explicit’ is very different from the photo search features where you can look for cats,” Doffman warns, “this is why we’re seeing serious concerns around false results and unintended consequences.”
"Most of the ML technology that is successfully implemented use the Naive Bayes algorithm"Dr Kesavan
A shift from classifiers to interpretations and scope creep enters the equation. “It’s the same in video where we are seeing the shift from ‘intrusion’ to ‘fighting’ or ‘violence’ and it’s remarkably difficult for ML to be entirely accurate without vast amounts of training data,” Doffman concludes.
The Adversarial Attack Paradox
What, then, if that training data is polluted? Adversarial ML attacks can feed deceptive inputs into models unnoticed by human trainers. “Adapting robust computer vision models to keep them safe from adversarial attacks,” Dr Kesavan says, “is [an ongoing] process and one that organizations should adapt continuously.” However, adding ‘noise’ to images allows an adversarial training method to reduce classification errors; it’s an adversarial attack paradox if you like. “As part of defense distillation,” Dr Kesavan explains, “the target model is used as the basis for a smaller model that results in a smoother output surface than the target model.” While it is plausible that the ML systems behind the detection of harmful content could be subject to adversarial attack, Dr Kazerounian thinks it unlikely that anyone is at the point of exercising these techniques in any broad way. “The return on investment on uploading offensive or harmful images that have been iteratively manipulated is likely to lead to your account getting banned before you ever find an instance of an adversarial attack that slips by the ML systems,” Dr Kazerounian tells Infosecurity.
Vahid Behzadan, Assistant Professor in Computer Science and Data Science at the Tagliatela College of Engineering, University of New Haven, isn’t so convinced. His lab has recently developed an attack methodology for fooling the deep learning models used for automated threat detection in the Twitter stream and investigating the adversarial manipulation of ML models for community detection in social networks. “Due to the limited generalizability of the current content moderation models,” Behzadan warns, “users and malicious actors can often find simple techniques for gaming and manipulating automated tools. This includes adversarial perturbations of text, multi-media content and even the social graph.”
Professor Lisa Short, chief research officer at the Global Foundation for Cyber Studies & Research in Washington DC, argues that, at a more foundational level, “if the data subsets were authenticated and assured at their entry point via the use of blockchain, it would drive the veracity and trust in deep neural networks of unsupervised ML.” Without it, Short concludes, “adversarial attacks are not just possible, but likely!”
Threat Benefit vs. Privacy Intrusion Conundrum
Perhaps a more significant threat to the future of ML within this context of harmful or misleading content is highlighted by the Apple debate: undoubted benefits set against the potential for misuse. It’s essential to make clear that the Apple announcement actually encompassed several different technologies. ML within the context of iMessage content on-device, rather than iCloud ‘hash and match’ of CSAM images, got somewhat lost in poorly communicated press releases. However, as Kevin Curran, senior IEEE member and Professor of Cybersecurity at Ulster University, warns, “the larger privacy debate revolves around a perceived intrusion by users where Apple is seen to be overreaching into items on our phone, which up to now, were never scanned.” Doffman agrees, telling us that “we need to bring users with us, and not risk the kind of backlash that has just hit Apple.”
“If the data subsets were authenticated and assured at their entry point via the use of blockchain, it would drive the veracity and trust in deep neural networks of unsupervised ML"Professor Lisa Short
Doffman confidently expects “substantial year-on-year improvements” and platforms can continue to train the ML systems. However, “we need wider debates as to what we’re trying to achieve,” he insists, “Apple fell flat with its iMessage ‘sexually explicit’ filter for this reason.”
When it comes to on-device screening, Doffman feels that’s going too far. It’s “the imbalance of invading the privacy of everyone to potentially catch a very tiny minority,” he says, “especially where that very tiny minority can take mitigating actions to evade detection.”
Dr Kazerounian agrees that the privacy concerns related to allowing automated surveillance of private data are real, even if used ostensibly to detect universally agreed-upon harmful content. “We can easily imagine repressive governments around the world deciding to use such automation for anything ranging from the persecution of the LGBTQ+ community to arbitrarily deciding political dissidents are engaged in harmful and subversive behavior,” he tells Infosecurity.
Yet, while Vahid Behzadan shares concerns regarding the misuse of AI for mass surveillance, he doesn’t “find the pursuit of privacy and civil liberties to be in opposition to the objectives of automated content moderation.” On the contrary, he says, “I find the two to be firmly aligned and mutually reinforcing. Without automated data-driven tools for detection and correction of false information, society would remain critically vulnerable to mass-manipulation via organized or emergent dissemination of misinformation.”
However, according to Nicola Whiting, MBE, CSO at Titania, this only addresses the technical problems. “In many ways, the ethical challenges are even more complex. If law enforcement wants to use ML to help scan for CSAM to identify abusers and protect the vulnerable, at some stage, those systems will need a dataset to learn from. Would using those images, even for a ‘good purpose,’ be ethical?” One thing for sure is that this is a debate that will play out for a long time to come.
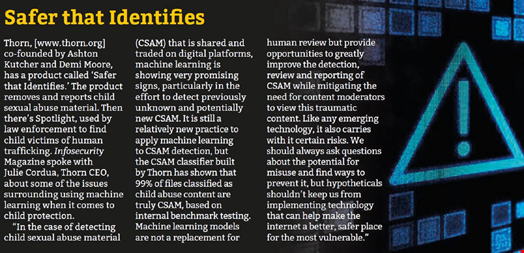